Thoughts & Ideas
Dec 1, 2023
Adding Generative AI to your Digital Product
Adding Generative AI to your Digital Product

Maca Balparda
Guest Author
How do you evaluate if Generative AI is something you should add to your solution?
In the fast-paced software ecosystem, Generative AI is a game-changer. But first, let’s explain what it actually is. GenAI is a subfield of Artificial Intelligence that focuses on developing systems that generate new content or data. It involves training machine learning models to learn patterns from existing data and use that knowledge to create unique, original output. This powerful technology promises to transform every industry by enabling machines to develop, learn, and generate new content.
With Light-it's expertise in the healthcare industry, we hope to give readers new perspectives on the possibilities of generative AI; many apply to any field, and many others are assessed from the Healthcare industry's point of view. We'll examine how to determine whether GenAI is a good fit for a particular project and its advantages in digital product development.
When is Generative AI suitable for a digital product?
While generative AI offers numerous benefits, assessing its suitability for a specific industry, such as healthcare, is crucial. Here are three factors to consider:
1. Data Availability
For generative AI to produce valuable outputs, there must be a large amount of high-quality training data. Check to see if you have access to a big enough, diverse dataset to support generative AI model training.
High-Quality Data
High-quality data refers to information that is accurate, reliable, and relevant. In the context of generative AI, data should be free from errors, biases, and inconsistencies. It should represent the real-world scenarios or concepts the AI model intends to understand and generate. For example, if the AI is trained to generate human-like text, the training data should consist of well-written, grammatically correct, and contextually meaningful text.
Example of a high-quality dataset in Healthcare:
Accurate and reliable information about patients' medical history, diagnoses, treatments, and outcomes. It ensures that electronic health records (EHRs) are free from errors, inconsistencies, and omissions. For example, if an AI model is designed to assist in diagnosing diseases based on medical records, high-quality data would include well-documented patient information with accurate lab results, clear diagnostic codes, and comprehensive treatment histories.
Large Amount of Data
The term "large amount" is relative and depends on the complexity of the task and the capacity of the model being trained. Generally, more data allows the model to learn a broader range of patterns and nuances. The size of the dataset is often measured in terms of the number of examples or instances. For complex tasks such as NLP (natural language processing) or image generation, training on millions or even billions of examples might be necessary to achieve optimal performance.
Example of a large dataset in Healthcare:
A large amount of data is crucial to train AI models effectively in healthcare. This could involve millions of patient records, medical imaging data, genomics data, and other relevant information. For instance, training a model to predict patient outcomes or personalize treatment plans might require a vast dataset that spans diverse populations, various medical conditions, and different healthcare settings. The large dataset helps the model learn patterns and correlations that can contribute to more accurate predictions and recommendations.
Diverse Dataset
A diverse dataset includes examples that cover various variations and scenarios relevant to the task. Diversity ensures that the model doesn't overfit to specific patterns present in the training data but generalizes well to new, unseen data. For generative AI, diversity might involve including examples from different sources, representing various perspectives, and covering a wide spectrum of input variations.
Example of a diverse dataset in healthcare:
A diverse dataset in healthcare would encompass a broad range of patient demographics, medical conditions, and healthcare scenarios. For example, if developing an AI system to recommend personalized treatment plans, the dataset should include diverse cases covering different age groups, genders, ethnicities, and medical conditions. This diversity ensures the model can generalize well to new and unseen patient cases, avoiding biases and limitations associated with a narrow dataset.
2. Problem Complexity
Consider the complexity of the problem your solution aims to address. While conventional machine learning techniques can yield satisfactory results for simpler problems, the use of generative AI may be necessary when dealing with intricate and diverse datasets.
Simple Problems:
1. Binary Classification
Example: Identifying whether a patient has a specific medical condition based on well-defined symptoms.
Why it's Simple: The task involves classifying patients into two categories (having or not having a condition) with clear symptom-based criteria.
2. Linear Regression
Example: Predicting a patient's blood pressure based on a single variable like age.
Why it's Simple: Assuming a linear relationship, predicting a continuous variable like blood pressure based on a single factor can be relatively straightforward.
Complex Problems:
1. Medical Image Analysis
Example: Detecting and classifying various abnormalities in radiology images (e.g., X-rays, MRIs).
Why it's Complex: Images can exhibit diverse pathologies, sizes, and locations, making it a complex task that requires understanding subtle visual patterns.
2. Clinical Natural Language Processing (NLP) - Extracting Insights from Medical Texts:
Example: Extracting information from unstructured clinical notes to assist in diagnosis or research.
Why it's Complex: Clinical notes often contain nuanced language, abbreviations, and references to a patient's complex medical history, requiring advanced language understanding.
3. Regulatory Considerations
Regulations regarding using artificial intelligence and generative AI (such as ChatGPT) in healthcare vary by country and region. It's important to note that the regulatory landscape is subject to change, and new guidelines are being introduced as these new technologies advance.
For a highly regulated domain such as digital health products, deploying generative AI in healthcare products may introduce additional regulatory considerations. Let’s break down the main 3.
1. Data Privacy Regulations: Health Insurance Portability and Accountability Act (HIPAA) in the United States; General Data Protection Regulation (GDPR) in the European Union.
What’s their purpose? These regulations focus on the protection of patients' sensitive health data. When using generative AI in healthcare, it's crucial to comply with data privacy regulations to ensure patient information's secure handling and storage.
2. Medical Device Regulations: CE marking in the EU; US Food and Drug Administration (FDA) regulations in the United States.
What’s their purpose? If the generative AI system is considered a medical device, it may need to adhere to specific regulatory requirements to ensure its safety and effectiveness. This is particularly important for AI systems involved in diagnosis, treatment planning, or decision-making.
3. Clinical Validation and Certification: FDA's Pre-Certification Program.
What’s their purpose?
Regulatory bodies may require evidence of the clinical validity and reliability of AI applications in healthcare. Pre-market certification or post-market surveillance processes may be in place to ensure the safety and efficacy of these applications.
Healthcare Generative AI: An Overview
Massive volumes of medical data, including electronic health records, medical imaging, and clinical notes, serve as the foundation for training generative AI algorithms. This enables them to generate synthetic data closely mirroring authentic patient information.
The power of generative AI lies in its ability to simulate and furnish precise data applicable across diverse tasks. This technology can augment limited datasets, generate synthetic patient profiles for simulation purposes, and formulate fresh patient records for training and validation. This empowers physicians and academics to delve into a broader spectrum of scenarios, experiment with concepts, and develop more refined models using generative AI.
Healthcare Generative AI: The Benefits
Given its many advantages, generative AI is an attractive method for healthcare solutions and provides immense benefits.
Privacy Preservation
Data must be anonymized and de-identified to adhere to privacy laws and safeguard patient confidentiality. With the assistance of generative AI, synthetic data can be produced that preserves the characteristics of the original patient data while excluding personally identifiable information. This artificial data can be used for research, training, and development without violating patient privacy.
Personalized Medicine
Because each patient is different, effective treatments for one person may not be effective for another. Generative AI can use patient-specific data to create individualized treatment plans and suggestions. By considering specific patient features, generative AI algorithms can aid in optimizing treatment choices and enhance patient outcomes.
Data Augmentation
The breadth and diversity of healthcare datasets are frequently constrained, which makes it difficult to develop reliable models. With the help of generative AI, current datasets can be supplemented with new data, expanding and diversifying it. This enlarged dataset can improve the performance and generalizability of machine learning models.
Testing and validation
The development of simulated scenarios for testing and evaluating healthcare products is possible by generative AI. Healthcare entrepreneurs can evaluate the efficacy and robustness of their algorithms and software solutions in diverse clinical contexts by producing synthetic patient data. This may even save time and resources during the development and testing phases.
Final thoughts
The potential of generative AI is immense. Combined with the right tech stack, digital product agency, and a thorough understanding of its capabilities, advantages, and suitable applications, you can make well-informed decisions that foster innovation and significantly enhance people's lives.
How do you evaluate if Generative AI is something you should add to your solution?
In the fast-paced software ecosystem, Generative AI is a game-changer. But first, let’s explain what it actually is. GenAI is a subfield of Artificial Intelligence that focuses on developing systems that generate new content or data. It involves training machine learning models to learn patterns from existing data and use that knowledge to create unique, original output. This powerful technology promises to transform every industry by enabling machines to develop, learn, and generate new content.
With Light-it's expertise in the healthcare industry, we hope to give readers new perspectives on the possibilities of generative AI; many apply to any field, and many others are assessed from the Healthcare industry's point of view. We'll examine how to determine whether GenAI is a good fit for a particular project and its advantages in digital product development.
When is Generative AI suitable for a digital product?
While generative AI offers numerous benefits, assessing its suitability for a specific industry, such as healthcare, is crucial. Here are three factors to consider:
1. Data Availability
For generative AI to produce valuable outputs, there must be a large amount of high-quality training data. Check to see if you have access to a big enough, diverse dataset to support generative AI model training.
High-Quality Data
High-quality data refers to information that is accurate, reliable, and relevant. In the context of generative AI, data should be free from errors, biases, and inconsistencies. It should represent the real-world scenarios or concepts the AI model intends to understand and generate. For example, if the AI is trained to generate human-like text, the training data should consist of well-written, grammatically correct, and contextually meaningful text.
Example of a high-quality dataset in Healthcare:
Accurate and reliable information about patients' medical history, diagnoses, treatments, and outcomes. It ensures that electronic health records (EHRs) are free from errors, inconsistencies, and omissions. For example, if an AI model is designed to assist in diagnosing diseases based on medical records, high-quality data would include well-documented patient information with accurate lab results, clear diagnostic codes, and comprehensive treatment histories.
Large Amount of Data
The term "large amount" is relative and depends on the complexity of the task and the capacity of the model being trained. Generally, more data allows the model to learn a broader range of patterns and nuances. The size of the dataset is often measured in terms of the number of examples or instances. For complex tasks such as NLP (natural language processing) or image generation, training on millions or even billions of examples might be necessary to achieve optimal performance.
Example of a large dataset in Healthcare:
A large amount of data is crucial to train AI models effectively in healthcare. This could involve millions of patient records, medical imaging data, genomics data, and other relevant information. For instance, training a model to predict patient outcomes or personalize treatment plans might require a vast dataset that spans diverse populations, various medical conditions, and different healthcare settings. The large dataset helps the model learn patterns and correlations that can contribute to more accurate predictions and recommendations.
Diverse Dataset
A diverse dataset includes examples that cover various variations and scenarios relevant to the task. Diversity ensures that the model doesn't overfit to specific patterns present in the training data but generalizes well to new, unseen data. For generative AI, diversity might involve including examples from different sources, representing various perspectives, and covering a wide spectrum of input variations.
Example of a diverse dataset in healthcare:
A diverse dataset in healthcare would encompass a broad range of patient demographics, medical conditions, and healthcare scenarios. For example, if developing an AI system to recommend personalized treatment plans, the dataset should include diverse cases covering different age groups, genders, ethnicities, and medical conditions. This diversity ensures the model can generalize well to new and unseen patient cases, avoiding biases and limitations associated with a narrow dataset.
2. Problem Complexity
Consider the complexity of the problem your solution aims to address. While conventional machine learning techniques can yield satisfactory results for simpler problems, the use of generative AI may be necessary when dealing with intricate and diverse datasets.
Simple Problems:
1. Binary Classification
Example: Identifying whether a patient has a specific medical condition based on well-defined symptoms.
Why it's Simple: The task involves classifying patients into two categories (having or not having a condition) with clear symptom-based criteria.
2. Linear Regression
Example: Predicting a patient's blood pressure based on a single variable like age.
Why it's Simple: Assuming a linear relationship, predicting a continuous variable like blood pressure based on a single factor can be relatively straightforward.
Complex Problems:
1. Medical Image Analysis
Example: Detecting and classifying various abnormalities in radiology images (e.g., X-rays, MRIs).
Why it's Complex: Images can exhibit diverse pathologies, sizes, and locations, making it a complex task that requires understanding subtle visual patterns.
2. Clinical Natural Language Processing (NLP) - Extracting Insights from Medical Texts:
Example: Extracting information from unstructured clinical notes to assist in diagnosis or research.
Why it's Complex: Clinical notes often contain nuanced language, abbreviations, and references to a patient's complex medical history, requiring advanced language understanding.
3. Regulatory Considerations
Regulations regarding using artificial intelligence and generative AI (such as ChatGPT) in healthcare vary by country and region. It's important to note that the regulatory landscape is subject to change, and new guidelines are being introduced as these new technologies advance.
For a highly regulated domain such as digital health products, deploying generative AI in healthcare products may introduce additional regulatory considerations. Let’s break down the main 3.
1. Data Privacy Regulations: Health Insurance Portability and Accountability Act (HIPAA) in the United States; General Data Protection Regulation (GDPR) in the European Union.
What’s their purpose? These regulations focus on the protection of patients' sensitive health data. When using generative AI in healthcare, it's crucial to comply with data privacy regulations to ensure patient information's secure handling and storage.
2. Medical Device Regulations: CE marking in the EU; US Food and Drug Administration (FDA) regulations in the United States.
What’s their purpose? If the generative AI system is considered a medical device, it may need to adhere to specific regulatory requirements to ensure its safety and effectiveness. This is particularly important for AI systems involved in diagnosis, treatment planning, or decision-making.
3. Clinical Validation and Certification: FDA's Pre-Certification Program.
What’s their purpose?
Regulatory bodies may require evidence of the clinical validity and reliability of AI applications in healthcare. Pre-market certification or post-market surveillance processes may be in place to ensure the safety and efficacy of these applications.
Healthcare Generative AI: An Overview
Massive volumes of medical data, including electronic health records, medical imaging, and clinical notes, serve as the foundation for training generative AI algorithms. This enables them to generate synthetic data closely mirroring authentic patient information.
The power of generative AI lies in its ability to simulate and furnish precise data applicable across diverse tasks. This technology can augment limited datasets, generate synthetic patient profiles for simulation purposes, and formulate fresh patient records for training and validation. This empowers physicians and academics to delve into a broader spectrum of scenarios, experiment with concepts, and develop more refined models using generative AI.
Healthcare Generative AI: The Benefits
Given its many advantages, generative AI is an attractive method for healthcare solutions and provides immense benefits.
Privacy Preservation
Data must be anonymized and de-identified to adhere to privacy laws and safeguard patient confidentiality. With the assistance of generative AI, synthetic data can be produced that preserves the characteristics of the original patient data while excluding personally identifiable information. This artificial data can be used for research, training, and development without violating patient privacy.
Personalized Medicine
Because each patient is different, effective treatments for one person may not be effective for another. Generative AI can use patient-specific data to create individualized treatment plans and suggestions. By considering specific patient features, generative AI algorithms can aid in optimizing treatment choices and enhance patient outcomes.
Data Augmentation
The breadth and diversity of healthcare datasets are frequently constrained, which makes it difficult to develop reliable models. With the help of generative AI, current datasets can be supplemented with new data, expanding and diversifying it. This enlarged dataset can improve the performance and generalizability of machine learning models.
Testing and validation
The development of simulated scenarios for testing and evaluating healthcare products is possible by generative AI. Healthcare entrepreneurs can evaluate the efficacy and robustness of their algorithms and software solutions in diverse clinical contexts by producing synthetic patient data. This may even save time and resources during the development and testing phases.
Final thoughts
The potential of generative AI is immense. Combined with the right tech stack, digital product agency, and a thorough understanding of its capabilities, advantages, and suitable applications, you can make well-informed decisions that foster innovation and significantly enhance people's lives.
How do you evaluate if Generative AI is something you should add to your solution?
In the fast-paced software ecosystem, Generative AI is a game-changer. But first, let’s explain what it actually is. GenAI is a subfield of Artificial Intelligence that focuses on developing systems that generate new content or data. It involves training machine learning models to learn patterns from existing data and use that knowledge to create unique, original output. This powerful technology promises to transform every industry by enabling machines to develop, learn, and generate new content.
With Light-it's expertise in the healthcare industry, we hope to give readers new perspectives on the possibilities of generative AI; many apply to any field, and many others are assessed from the Healthcare industry's point of view. We'll examine how to determine whether GenAI is a good fit for a particular project and its advantages in digital product development.
When is Generative AI suitable for a digital product?
While generative AI offers numerous benefits, assessing its suitability for a specific industry, such as healthcare, is crucial. Here are three factors to consider:
1. Data Availability
For generative AI to produce valuable outputs, there must be a large amount of high-quality training data. Check to see if you have access to a big enough, diverse dataset to support generative AI model training.
High-Quality Data
High-quality data refers to information that is accurate, reliable, and relevant. In the context of generative AI, data should be free from errors, biases, and inconsistencies. It should represent the real-world scenarios or concepts the AI model intends to understand and generate. For example, if the AI is trained to generate human-like text, the training data should consist of well-written, grammatically correct, and contextually meaningful text.
Example of a high-quality dataset in Healthcare:
Accurate and reliable information about patients' medical history, diagnoses, treatments, and outcomes. It ensures that electronic health records (EHRs) are free from errors, inconsistencies, and omissions. For example, if an AI model is designed to assist in diagnosing diseases based on medical records, high-quality data would include well-documented patient information with accurate lab results, clear diagnostic codes, and comprehensive treatment histories.
Large Amount of Data
The term "large amount" is relative and depends on the complexity of the task and the capacity of the model being trained. Generally, more data allows the model to learn a broader range of patterns and nuances. The size of the dataset is often measured in terms of the number of examples or instances. For complex tasks such as NLP (natural language processing) or image generation, training on millions or even billions of examples might be necessary to achieve optimal performance.
Example of a large dataset in Healthcare:
A large amount of data is crucial to train AI models effectively in healthcare. This could involve millions of patient records, medical imaging data, genomics data, and other relevant information. For instance, training a model to predict patient outcomes or personalize treatment plans might require a vast dataset that spans diverse populations, various medical conditions, and different healthcare settings. The large dataset helps the model learn patterns and correlations that can contribute to more accurate predictions and recommendations.
Diverse Dataset
A diverse dataset includes examples that cover various variations and scenarios relevant to the task. Diversity ensures that the model doesn't overfit to specific patterns present in the training data but generalizes well to new, unseen data. For generative AI, diversity might involve including examples from different sources, representing various perspectives, and covering a wide spectrum of input variations.
Example of a diverse dataset in healthcare:
A diverse dataset in healthcare would encompass a broad range of patient demographics, medical conditions, and healthcare scenarios. For example, if developing an AI system to recommend personalized treatment plans, the dataset should include diverse cases covering different age groups, genders, ethnicities, and medical conditions. This diversity ensures the model can generalize well to new and unseen patient cases, avoiding biases and limitations associated with a narrow dataset.
2. Problem Complexity
Consider the complexity of the problem your solution aims to address. While conventional machine learning techniques can yield satisfactory results for simpler problems, the use of generative AI may be necessary when dealing with intricate and diverse datasets.
Simple Problems:
1. Binary Classification
Example: Identifying whether a patient has a specific medical condition based on well-defined symptoms.
Why it's Simple: The task involves classifying patients into two categories (having or not having a condition) with clear symptom-based criteria.
2. Linear Regression
Example: Predicting a patient's blood pressure based on a single variable like age.
Why it's Simple: Assuming a linear relationship, predicting a continuous variable like blood pressure based on a single factor can be relatively straightforward.
Complex Problems:
1. Medical Image Analysis
Example: Detecting and classifying various abnormalities in radiology images (e.g., X-rays, MRIs).
Why it's Complex: Images can exhibit diverse pathologies, sizes, and locations, making it a complex task that requires understanding subtle visual patterns.
2. Clinical Natural Language Processing (NLP) - Extracting Insights from Medical Texts:
Example: Extracting information from unstructured clinical notes to assist in diagnosis or research.
Why it's Complex: Clinical notes often contain nuanced language, abbreviations, and references to a patient's complex medical history, requiring advanced language understanding.
3. Regulatory Considerations
Regulations regarding using artificial intelligence and generative AI (such as ChatGPT) in healthcare vary by country and region. It's important to note that the regulatory landscape is subject to change, and new guidelines are being introduced as these new technologies advance.
For a highly regulated domain such as digital health products, deploying generative AI in healthcare products may introduce additional regulatory considerations. Let’s break down the main 3.
1. Data Privacy Regulations: Health Insurance Portability and Accountability Act (HIPAA) in the United States; General Data Protection Regulation (GDPR) in the European Union.
What’s their purpose? These regulations focus on the protection of patients' sensitive health data. When using generative AI in healthcare, it's crucial to comply with data privacy regulations to ensure patient information's secure handling and storage.
2. Medical Device Regulations: CE marking in the EU; US Food and Drug Administration (FDA) regulations in the United States.
What’s their purpose? If the generative AI system is considered a medical device, it may need to adhere to specific regulatory requirements to ensure its safety and effectiveness. This is particularly important for AI systems involved in diagnosis, treatment planning, or decision-making.
3. Clinical Validation and Certification: FDA's Pre-Certification Program.
What’s their purpose?
Regulatory bodies may require evidence of the clinical validity and reliability of AI applications in healthcare. Pre-market certification or post-market surveillance processes may be in place to ensure the safety and efficacy of these applications.
Healthcare Generative AI: An Overview
Massive volumes of medical data, including electronic health records, medical imaging, and clinical notes, serve as the foundation for training generative AI algorithms. This enables them to generate synthetic data closely mirroring authentic patient information.
The power of generative AI lies in its ability to simulate and furnish precise data applicable across diverse tasks. This technology can augment limited datasets, generate synthetic patient profiles for simulation purposes, and formulate fresh patient records for training and validation. This empowers physicians and academics to delve into a broader spectrum of scenarios, experiment with concepts, and develop more refined models using generative AI.
Healthcare Generative AI: The Benefits
Given its many advantages, generative AI is an attractive method for healthcare solutions and provides immense benefits.
Privacy Preservation
Data must be anonymized and de-identified to adhere to privacy laws and safeguard patient confidentiality. With the assistance of generative AI, synthetic data can be produced that preserves the characteristics of the original patient data while excluding personally identifiable information. This artificial data can be used for research, training, and development without violating patient privacy.
Personalized Medicine
Because each patient is different, effective treatments for one person may not be effective for another. Generative AI can use patient-specific data to create individualized treatment plans and suggestions. By considering specific patient features, generative AI algorithms can aid in optimizing treatment choices and enhance patient outcomes.
Data Augmentation
The breadth and diversity of healthcare datasets are frequently constrained, which makes it difficult to develop reliable models. With the help of generative AI, current datasets can be supplemented with new data, expanding and diversifying it. This enlarged dataset can improve the performance and generalizability of machine learning models.
Testing and validation
The development of simulated scenarios for testing and evaluating healthcare products is possible by generative AI. Healthcare entrepreneurs can evaluate the efficacy and robustness of their algorithms and software solutions in diverse clinical contexts by producing synthetic patient data. This may even save time and resources during the development and testing phases.
Final thoughts
The potential of generative AI is immense. Combined with the right tech stack, digital product agency, and a thorough understanding of its capabilities, advantages, and suitable applications, you can make well-informed decisions that foster innovation and significantly enhance people's lives.
How do you evaluate if Generative AI is something you should add to your solution?
In the fast-paced software ecosystem, Generative AI is a game-changer. But first, let’s explain what it actually is. GenAI is a subfield of Artificial Intelligence that focuses on developing systems that generate new content or data. It involves training machine learning models to learn patterns from existing data and use that knowledge to create unique, original output. This powerful technology promises to transform every industry by enabling machines to develop, learn, and generate new content.
With Light-it's expertise in the healthcare industry, we hope to give readers new perspectives on the possibilities of generative AI; many apply to any field, and many others are assessed from the Healthcare industry's point of view. We'll examine how to determine whether GenAI is a good fit for a particular project and its advantages in digital product development.
When is Generative AI suitable for a digital product?
While generative AI offers numerous benefits, assessing its suitability for a specific industry, such as healthcare, is crucial. Here are three factors to consider:
1. Data Availability
For generative AI to produce valuable outputs, there must be a large amount of high-quality training data. Check to see if you have access to a big enough, diverse dataset to support generative AI model training.
High-Quality Data
High-quality data refers to information that is accurate, reliable, and relevant. In the context of generative AI, data should be free from errors, biases, and inconsistencies. It should represent the real-world scenarios or concepts the AI model intends to understand and generate. For example, if the AI is trained to generate human-like text, the training data should consist of well-written, grammatically correct, and contextually meaningful text.
Example of a high-quality dataset in Healthcare:
Accurate and reliable information about patients' medical history, diagnoses, treatments, and outcomes. It ensures that electronic health records (EHRs) are free from errors, inconsistencies, and omissions. For example, if an AI model is designed to assist in diagnosing diseases based on medical records, high-quality data would include well-documented patient information with accurate lab results, clear diagnostic codes, and comprehensive treatment histories.
Large Amount of Data
The term "large amount" is relative and depends on the complexity of the task and the capacity of the model being trained. Generally, more data allows the model to learn a broader range of patterns and nuances. The size of the dataset is often measured in terms of the number of examples or instances. For complex tasks such as NLP (natural language processing) or image generation, training on millions or even billions of examples might be necessary to achieve optimal performance.
Example of a large dataset in Healthcare:
A large amount of data is crucial to train AI models effectively in healthcare. This could involve millions of patient records, medical imaging data, genomics data, and other relevant information. For instance, training a model to predict patient outcomes or personalize treatment plans might require a vast dataset that spans diverse populations, various medical conditions, and different healthcare settings. The large dataset helps the model learn patterns and correlations that can contribute to more accurate predictions and recommendations.
Diverse Dataset
A diverse dataset includes examples that cover various variations and scenarios relevant to the task. Diversity ensures that the model doesn't overfit to specific patterns present in the training data but generalizes well to new, unseen data. For generative AI, diversity might involve including examples from different sources, representing various perspectives, and covering a wide spectrum of input variations.
Example of a diverse dataset in healthcare:
A diverse dataset in healthcare would encompass a broad range of patient demographics, medical conditions, and healthcare scenarios. For example, if developing an AI system to recommend personalized treatment plans, the dataset should include diverse cases covering different age groups, genders, ethnicities, and medical conditions. This diversity ensures the model can generalize well to new and unseen patient cases, avoiding biases and limitations associated with a narrow dataset.
2. Problem Complexity
Consider the complexity of the problem your solution aims to address. While conventional machine learning techniques can yield satisfactory results for simpler problems, the use of generative AI may be necessary when dealing with intricate and diverse datasets.
Simple Problems:
1. Binary Classification
Example: Identifying whether a patient has a specific medical condition based on well-defined symptoms.
Why it's Simple: The task involves classifying patients into two categories (having or not having a condition) with clear symptom-based criteria.
2. Linear Regression
Example: Predicting a patient's blood pressure based on a single variable like age.
Why it's Simple: Assuming a linear relationship, predicting a continuous variable like blood pressure based on a single factor can be relatively straightforward.
Complex Problems:
1. Medical Image Analysis
Example: Detecting and classifying various abnormalities in radiology images (e.g., X-rays, MRIs).
Why it's Complex: Images can exhibit diverse pathologies, sizes, and locations, making it a complex task that requires understanding subtle visual patterns.
2. Clinical Natural Language Processing (NLP) - Extracting Insights from Medical Texts:
Example: Extracting information from unstructured clinical notes to assist in diagnosis or research.
Why it's Complex: Clinical notes often contain nuanced language, abbreviations, and references to a patient's complex medical history, requiring advanced language understanding.
3. Regulatory Considerations
Regulations regarding using artificial intelligence and generative AI (such as ChatGPT) in healthcare vary by country and region. It's important to note that the regulatory landscape is subject to change, and new guidelines are being introduced as these new technologies advance.
For a highly regulated domain such as digital health products, deploying generative AI in healthcare products may introduce additional regulatory considerations. Let’s break down the main 3.
1. Data Privacy Regulations: Health Insurance Portability and Accountability Act (HIPAA) in the United States; General Data Protection Regulation (GDPR) in the European Union.
What’s their purpose? These regulations focus on the protection of patients' sensitive health data. When using generative AI in healthcare, it's crucial to comply with data privacy regulations to ensure patient information's secure handling and storage.
2. Medical Device Regulations: CE marking in the EU; US Food and Drug Administration (FDA) regulations in the United States.
What’s their purpose? If the generative AI system is considered a medical device, it may need to adhere to specific regulatory requirements to ensure its safety and effectiveness. This is particularly important for AI systems involved in diagnosis, treatment planning, or decision-making.
3. Clinical Validation and Certification: FDA's Pre-Certification Program.
What’s their purpose?
Regulatory bodies may require evidence of the clinical validity and reliability of AI applications in healthcare. Pre-market certification or post-market surveillance processes may be in place to ensure the safety and efficacy of these applications.
Healthcare Generative AI: An Overview
Massive volumes of medical data, including electronic health records, medical imaging, and clinical notes, serve as the foundation for training generative AI algorithms. This enables them to generate synthetic data closely mirroring authentic patient information.
The power of generative AI lies in its ability to simulate and furnish precise data applicable across diverse tasks. This technology can augment limited datasets, generate synthetic patient profiles for simulation purposes, and formulate fresh patient records for training and validation. This empowers physicians and academics to delve into a broader spectrum of scenarios, experiment with concepts, and develop more refined models using generative AI.
Healthcare Generative AI: The Benefits
Given its many advantages, generative AI is an attractive method for healthcare solutions and provides immense benefits.
Privacy Preservation
Data must be anonymized and de-identified to adhere to privacy laws and safeguard patient confidentiality. With the assistance of generative AI, synthetic data can be produced that preserves the characteristics of the original patient data while excluding personally identifiable information. This artificial data can be used for research, training, and development without violating patient privacy.
Personalized Medicine
Because each patient is different, effective treatments for one person may not be effective for another. Generative AI can use patient-specific data to create individualized treatment plans and suggestions. By considering specific patient features, generative AI algorithms can aid in optimizing treatment choices and enhance patient outcomes.
Data Augmentation
The breadth and diversity of healthcare datasets are frequently constrained, which makes it difficult to develop reliable models. With the help of generative AI, current datasets can be supplemented with new data, expanding and diversifying it. This enlarged dataset can improve the performance and generalizability of machine learning models.
Testing and validation
The development of simulated scenarios for testing and evaluating healthcare products is possible by generative AI. Healthcare entrepreneurs can evaluate the efficacy and robustness of their algorithms and software solutions in diverse clinical contexts by producing synthetic patient data. This may even save time and resources during the development and testing phases.
Final thoughts
The potential of generative AI is immense. Combined with the right tech stack, digital product agency, and a thorough understanding of its capabilities, advantages, and suitable applications, you can make well-informed decisions that foster innovation and significantly enhance people's lives.
Latest From Our Blog

Thoughts & Ideas
HIPAA on PaaS: Vercel, Render, Heroku, Railway, and Aptible

Gabriella Valdes
Customer Success

Thoughts & Ideas
HIPAA on PaaS: Vercel, Render, Heroku, Railway, and Aptible

Gabriella Valdes
Customer Success

Thoughts & Ideas
HIPAA on PaaS: Vercel, Render, Heroku, Railway, and Aptible

Gabriella Valdes
Customer Success

Thoughts & Ideas
HIPAA on PaaS: Vercel, Render, Heroku, Railway, and Aptible

Gabriella Valdes
Customer Success
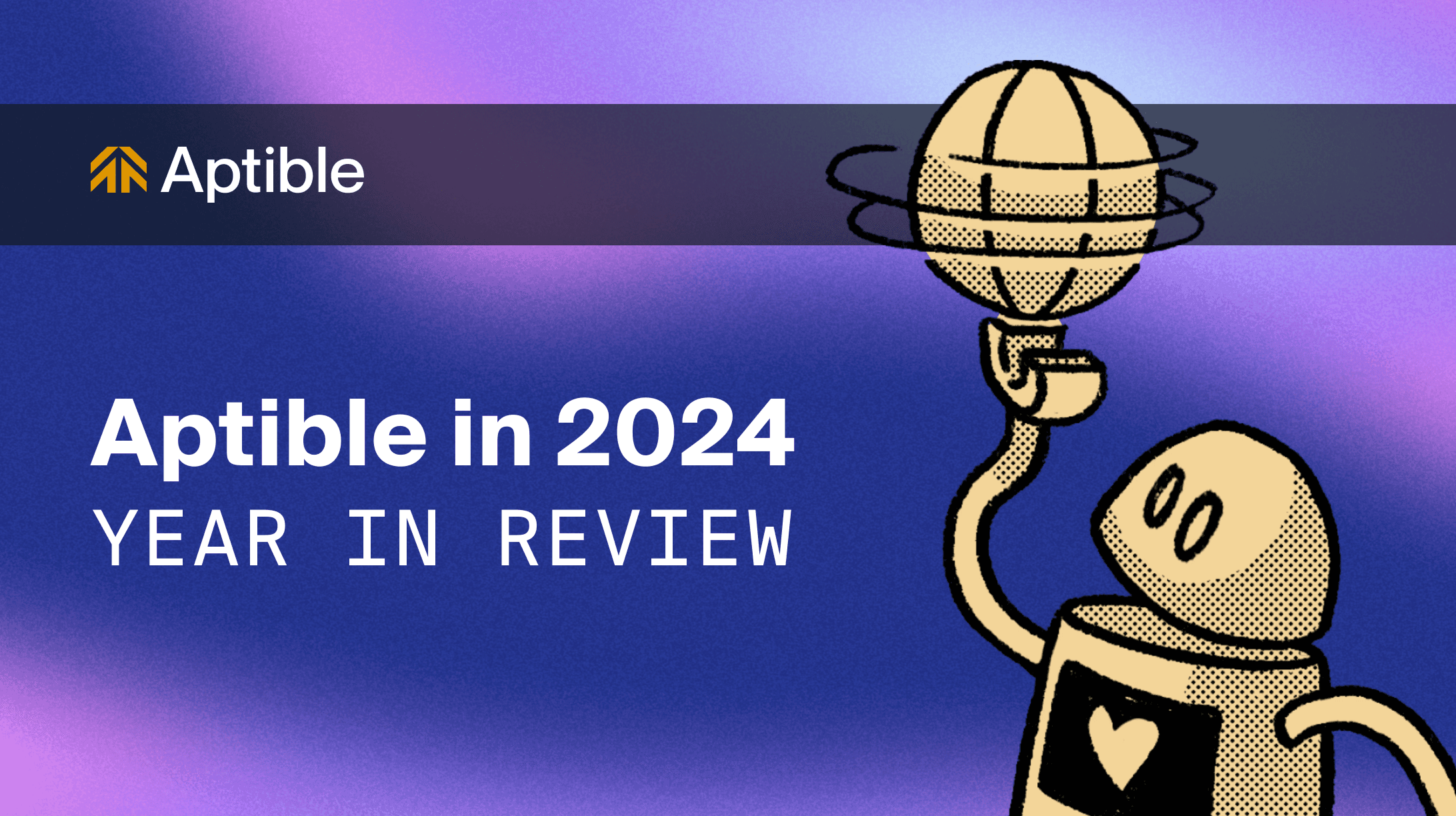
Company
Aptible in 2024: Year in Review
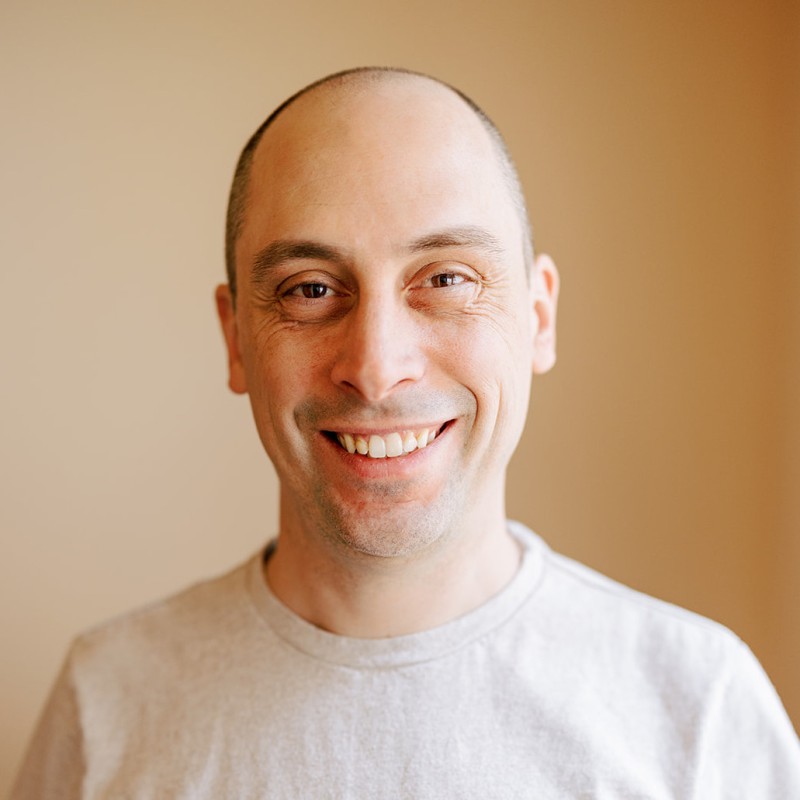
Frank Macreery
CEO
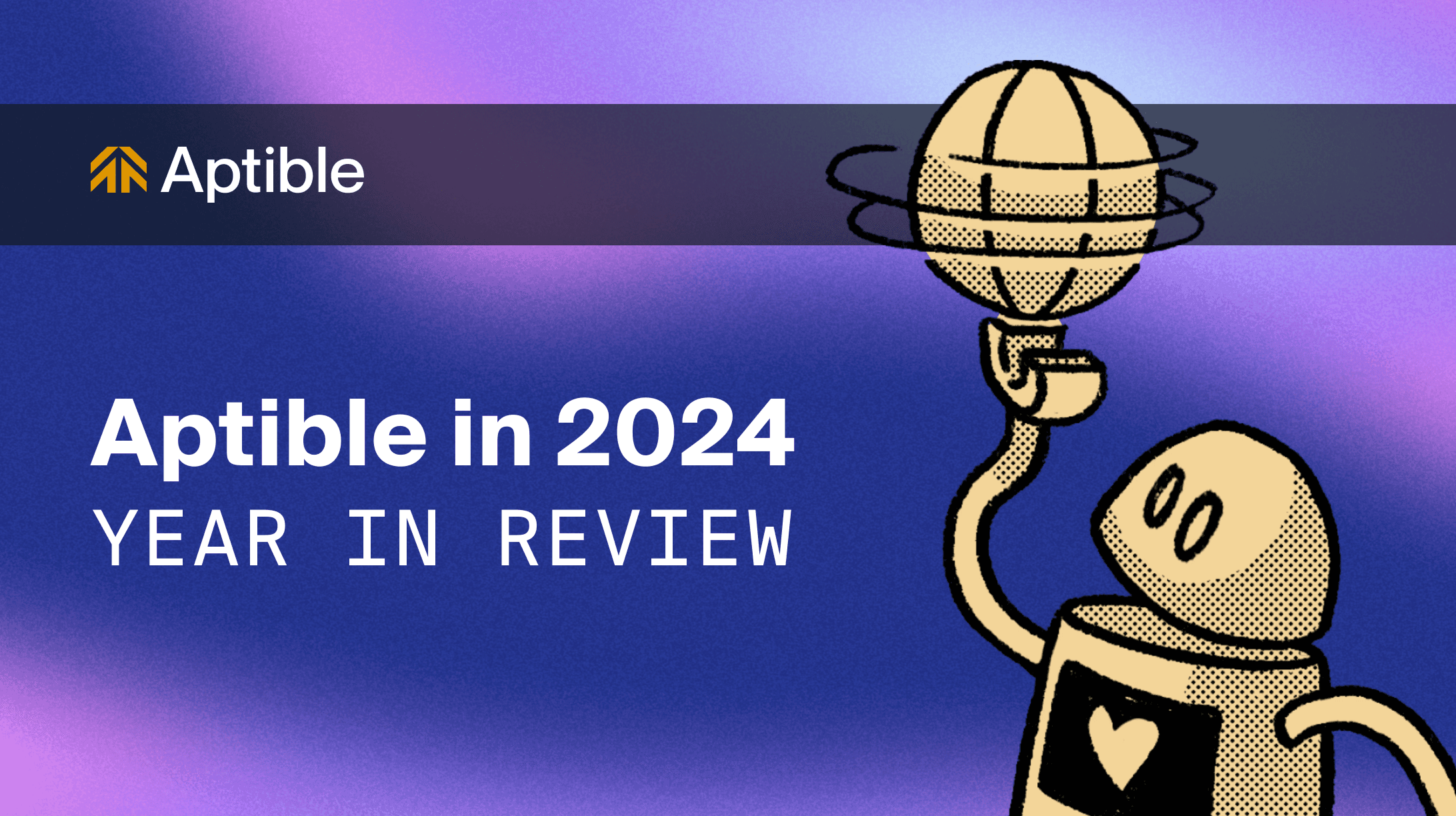
Company
Aptible in 2024: Year in Review
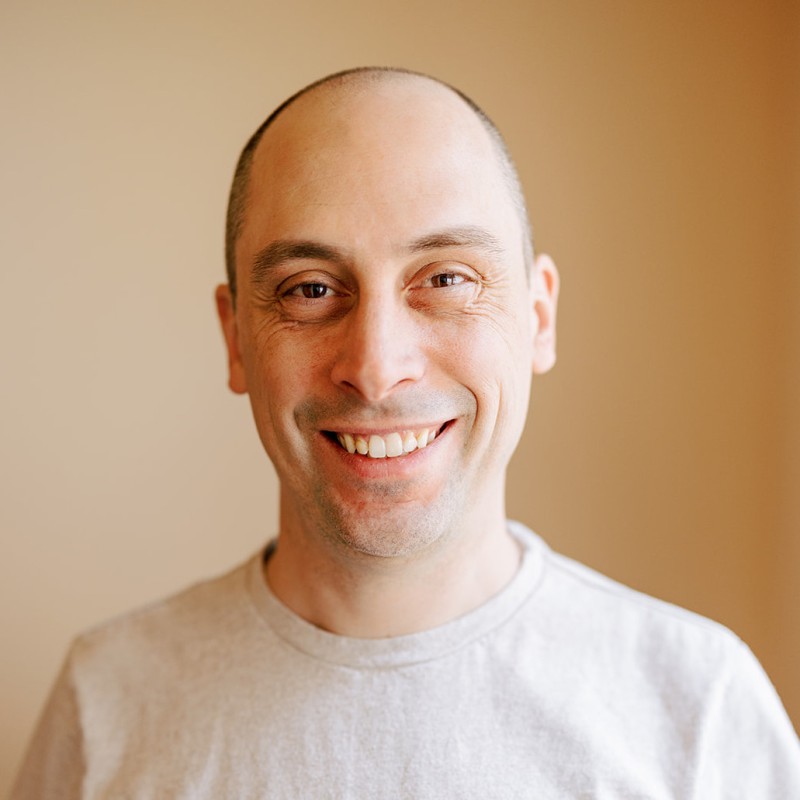
Frank Macreery
CEO
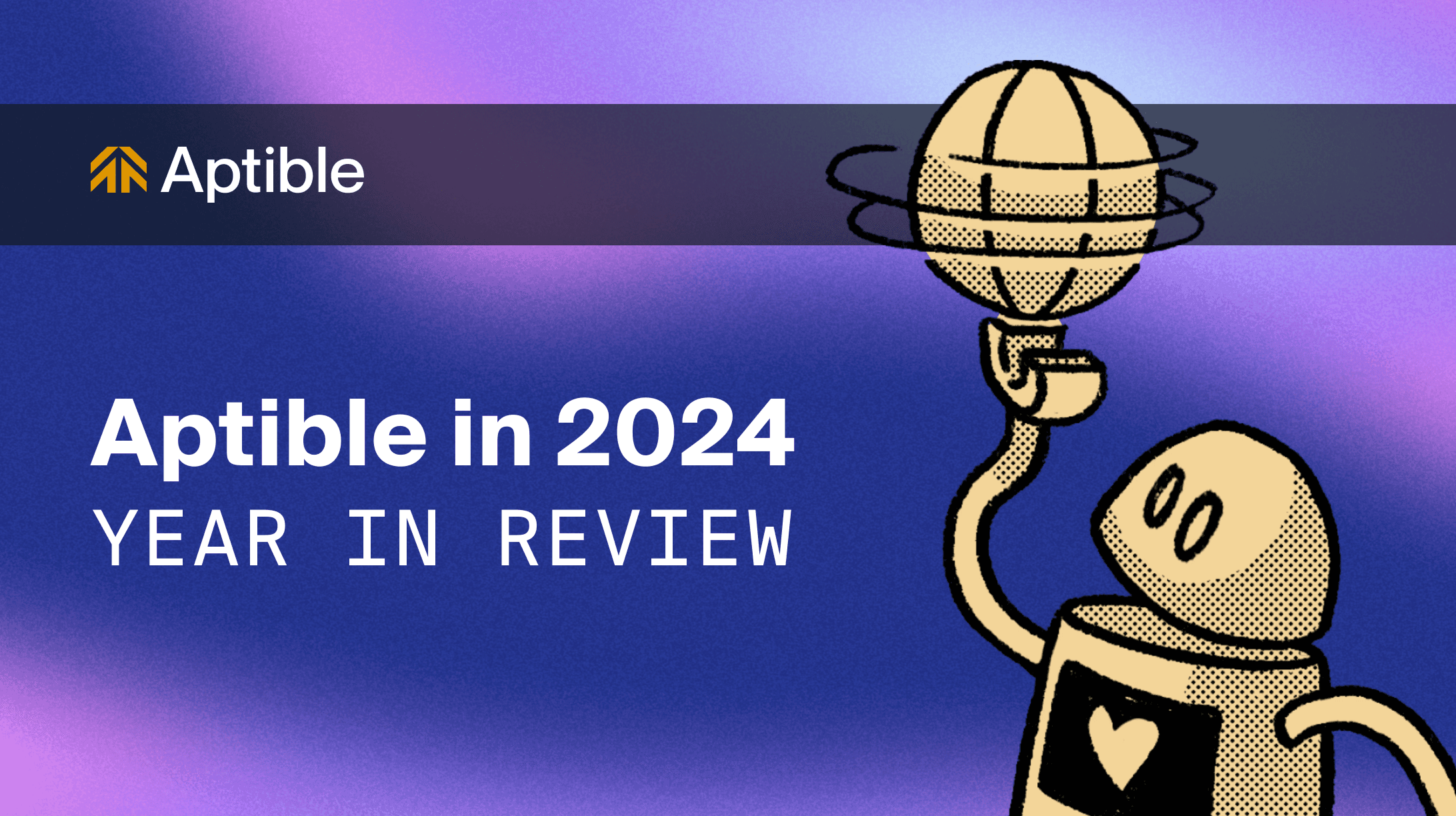
Company
Aptible in 2024: Year in Review
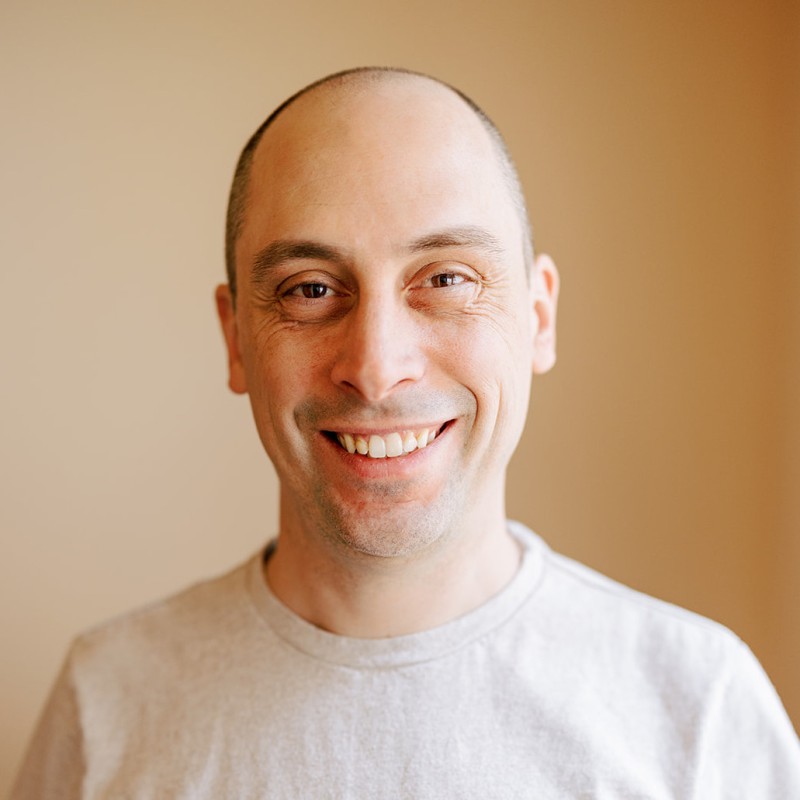
Frank Macreery
CEO
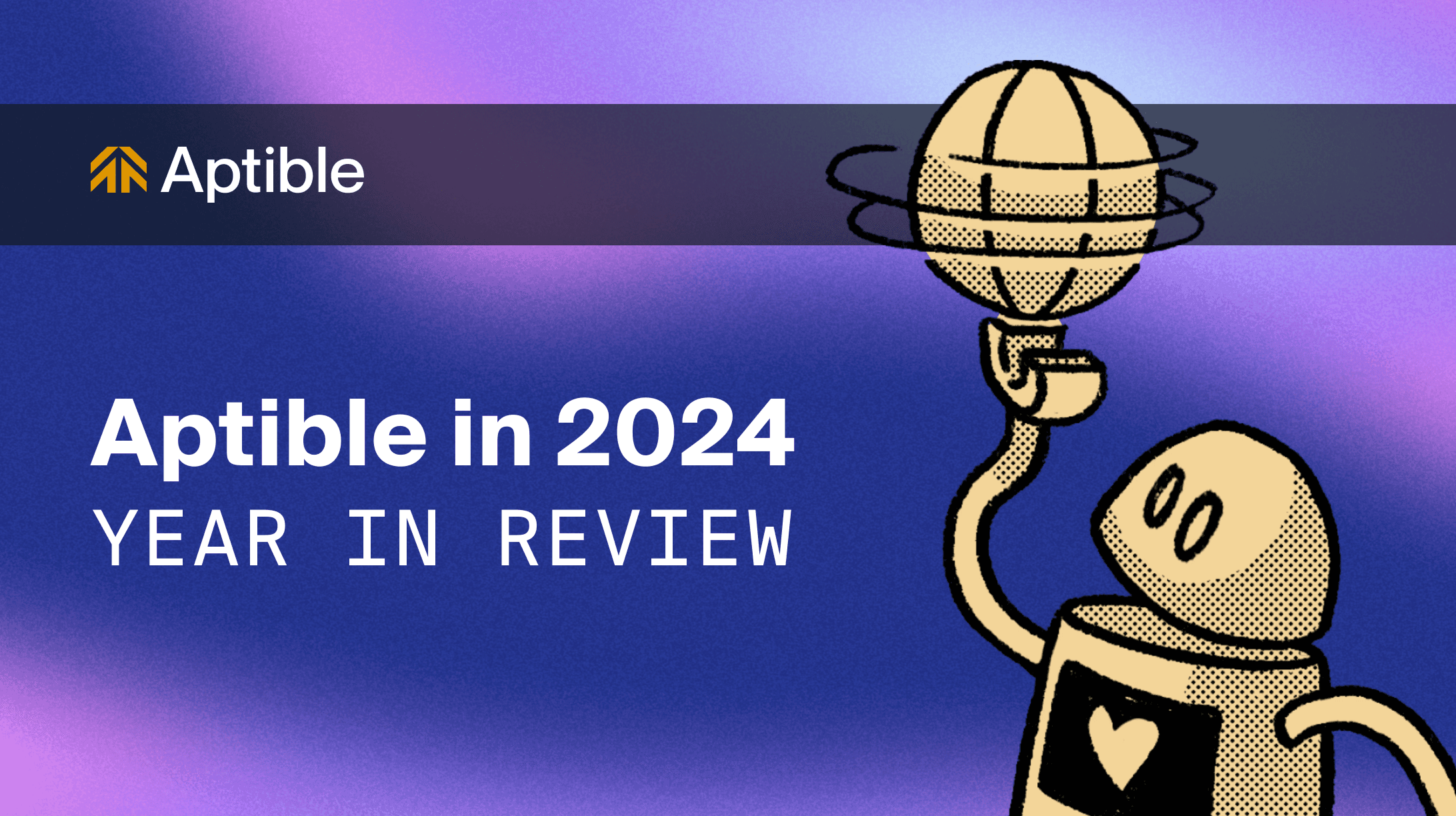
Company
Aptible in 2024: Year in Review
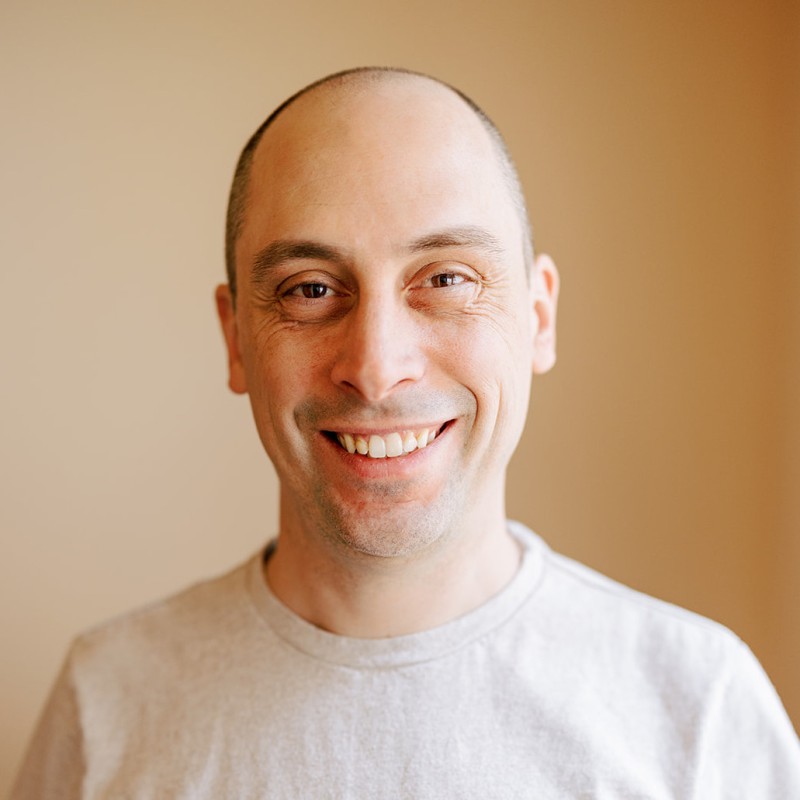
Frank Macreery
CEO
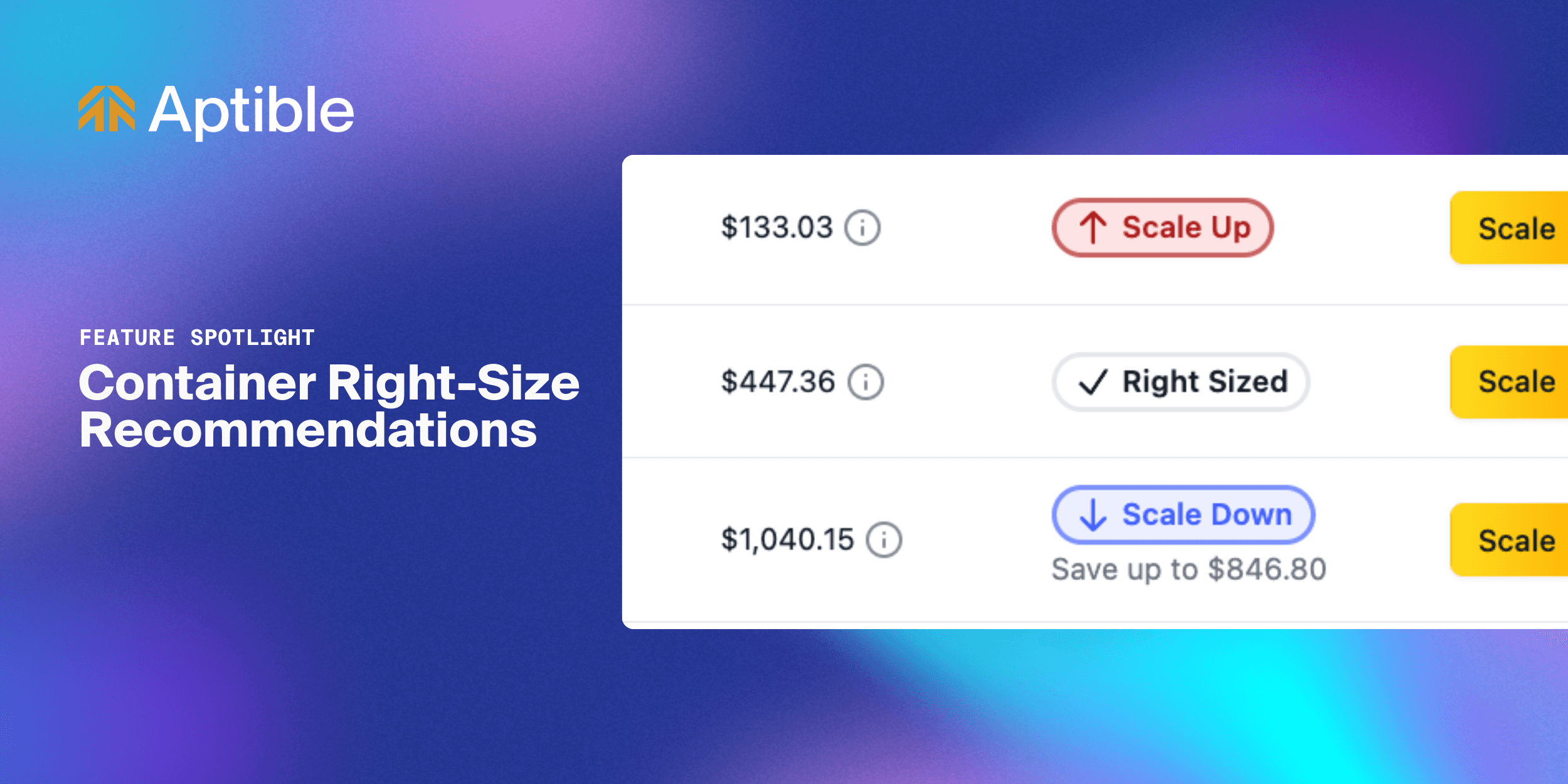
Changelog
Introducing Container Right-Size Recommendations for Apps and Databases

Gabriella Valdes
Customer Success
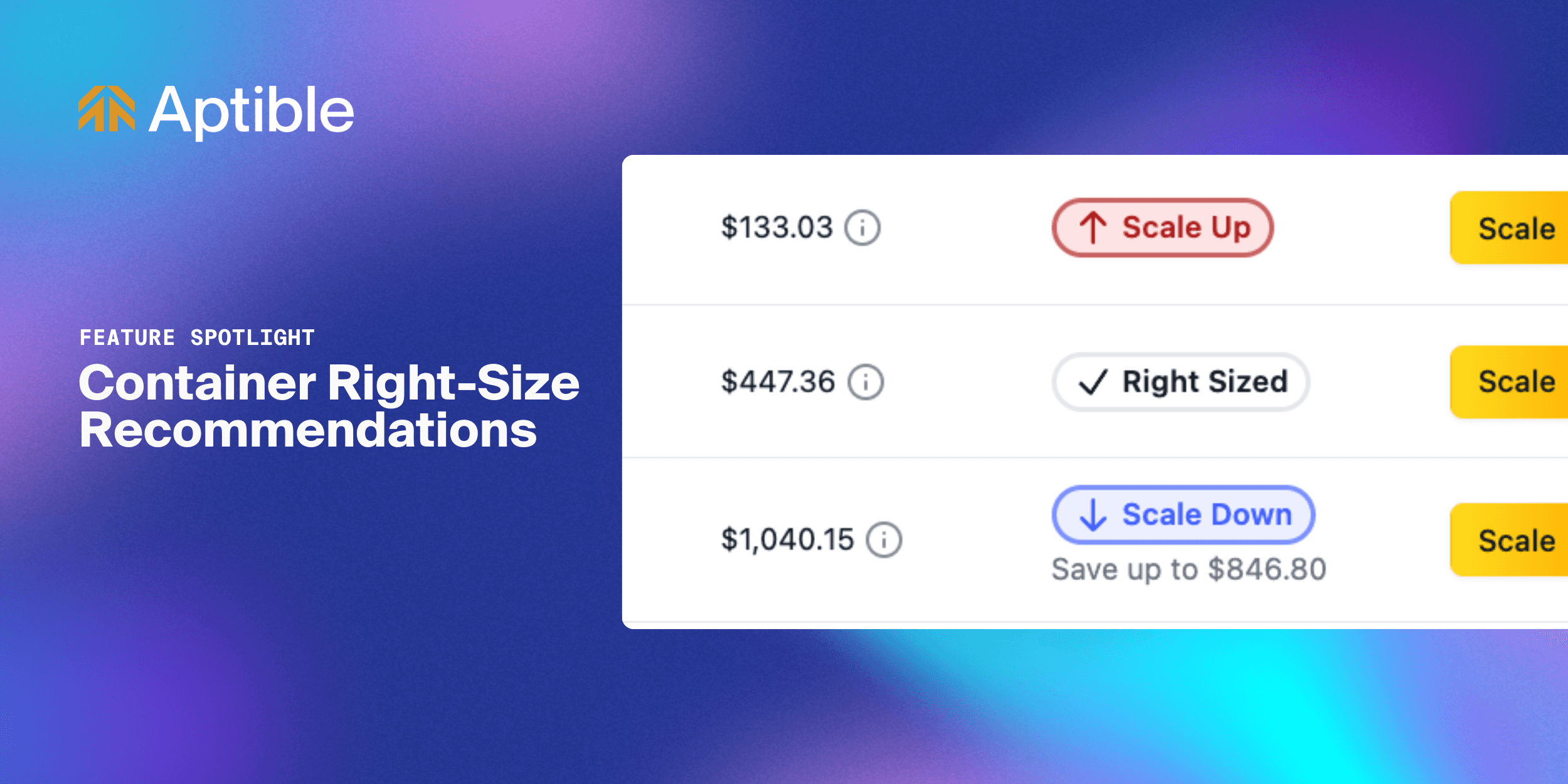
Changelog
Introducing Container Right-Size Recommendations for Apps and Databases

Gabriella Valdes
Customer Success
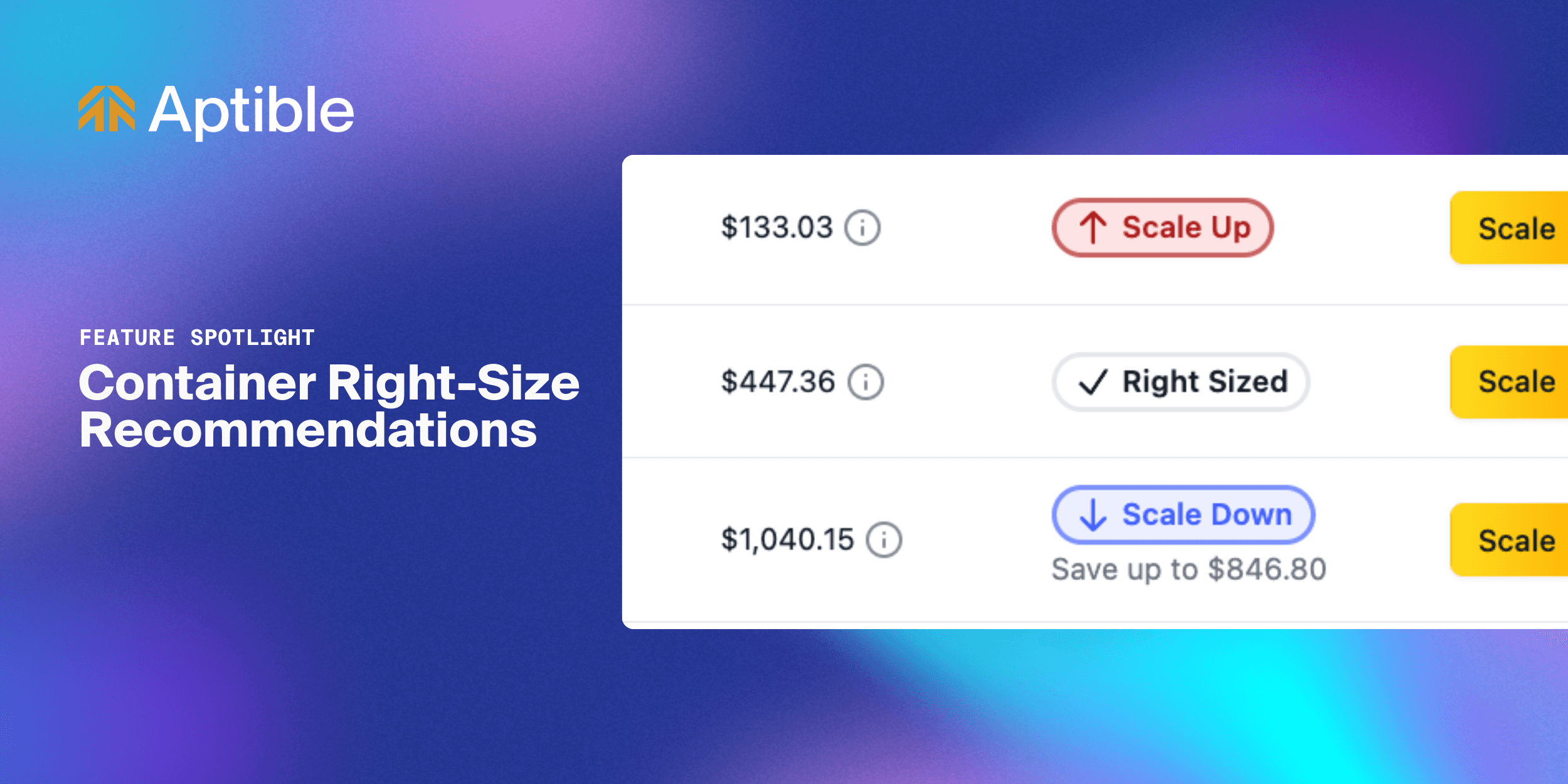
Changelog
Introducing Container Right-Size Recommendations for Apps and Databases

Gabriella Valdes
Customer Success
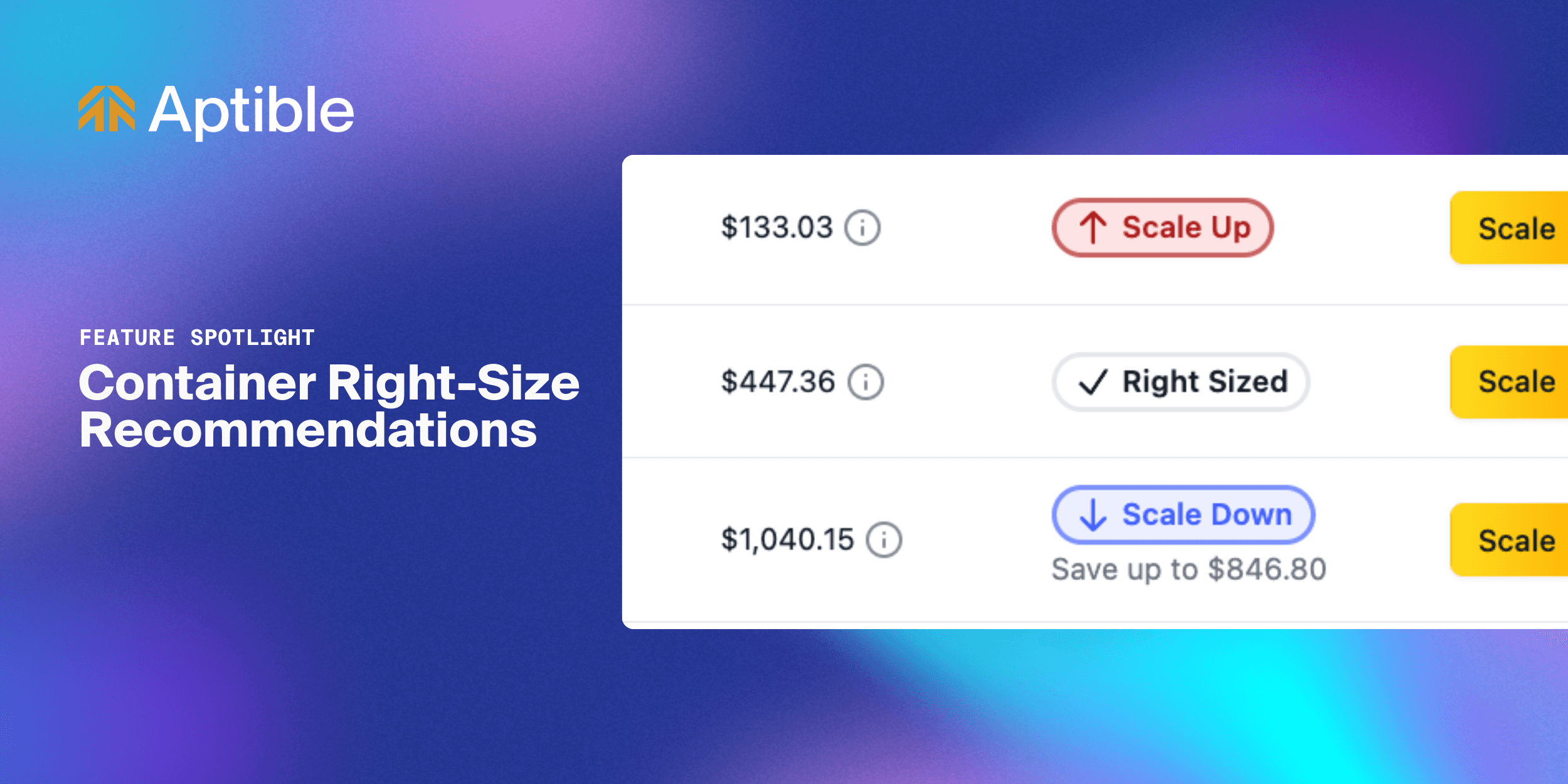
Changelog
Introducing Container Right-Size Recommendations for Apps and Databases

Gabriella Valdes
Customer Success




548 Market St #75826 San Francisco, CA 94104
© 2025. All rights reserved. Privacy Policy
548 Market St #75826 San Francisco, CA 94104
© 2025. All rights reserved. Privacy Policy
548 Market St #75826 San Francisco, CA 94104
© 2025. All rights reserved. Privacy Policy
548 Market St #75826 San Francisco, CA 94104
© 2025. All rights reserved. Privacy Policy